Speakers - Symposium 2023
Table of contents
Francesca Toma
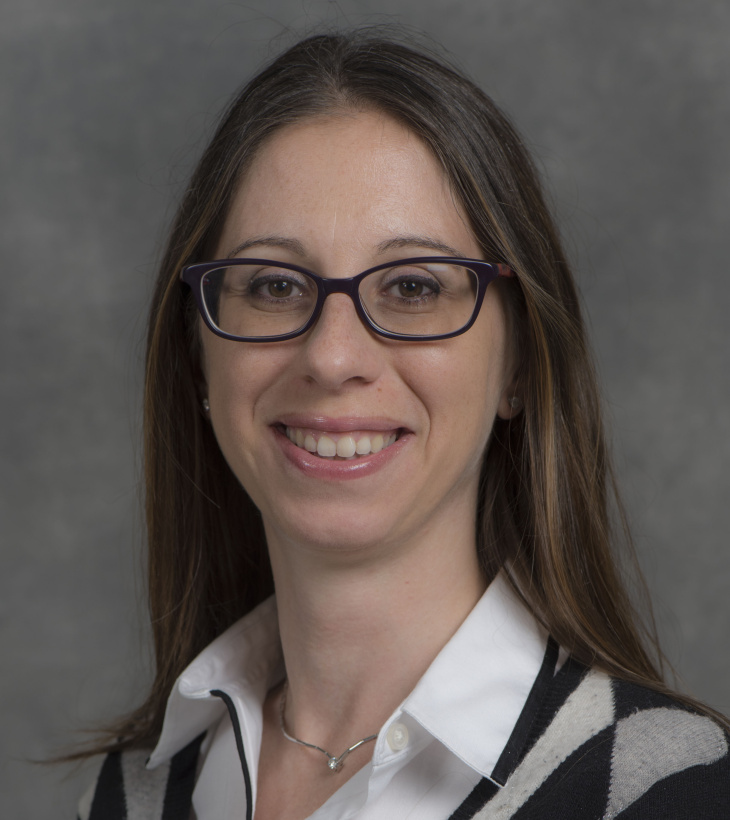
Helmholtz Zentrum Hereon / Helmut Schmidt University, DE
Prof. Toma is the Director of the Institute of Functional Materials for Sustainability at Helmholtz Zentrum Hereon and a Distinguished Helmholtz Professor at Helmut Schmidt University. Her research centers on the synthesis and characterization of sustainable materials for renewable energy and biological applications. She also holds a position as a Visiting Professor at Lawrence Berkeley National Laboratory.
She earned her Ph.D. in Biophysics from the International School of Advanced Studies in Italy in 2009, and acquired postdoctoral experience at the University of Trieste, before moving to the University of California, Santa Barbara as a Marie Curie Researcher in 2011, and to the University of California, Berkeley in 2013. For nearly a decade, she was as a Staff Scientist at Lawrence Berkeley National Lab, where she served as the Program lead of the Liquid Sunlight Alliance and as the Photoelectrochemistry Technology Lead for HydroGEN.
Prof. Toma's has co-authored 100 publications, focusing on (photo)electrocatalysis, drug delivery, and tissue engineering. Her work has garnered international recognition with several awards. She was honored as one of the "100 Women of Materials Science" by the Royal Society of Chemistry in 2018 and received the "Rising Star" Award from the American Chemical Society in 2021. She was also awarded the “Alfredo di Braccio Award” by the Italian Academy of Science. Her achievements culminated in her selection as an Oppenheimer Fellow by the US National Laboratory Directors' Council in 2022, underscoring her contributions as a leader to advancing scientific research.
Integrated Systems for Solar Fuel Production: Design and Characterization
Carbon neutral energy sources that are scalable, deployable, and cost effective will be required at an unprecedented scale to halt irreversible climate change. To design novel materials that can efficiently produce energy with minimal impact on the environment, few factors are of primary importance: i) complete understanding of the properties of the most selective and efficient reaction environments, and ii) correlative characterization of their behavior under operating conditions.
This talk will include a correlative characterization approach that provides nanoscale resolved functional and chemical information to explain materials stability for solar water splitting. In addition, it will also focus on the role played by microenvironments and on the opportunities offered by the utilization of sunlight for CO2 reduction.
Beatriz Roldán Cuenya
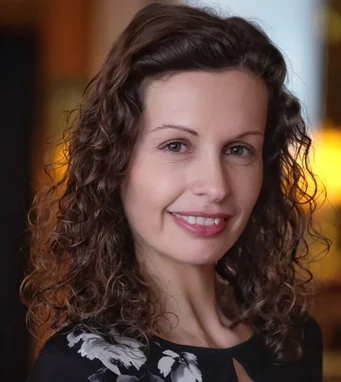
Department of Interface Science, Fritz Haber Institute of the Max Planck Society, DE
Beatriz Roldán Cuenya is a Professor and Director of the Interface Science Department of the Fritz-Haber Institute of the Max-Planck Society in Berlin Germany since 2017. She received her PhD in solid state physics from the University of Duisburg-Essen (Germany) in 2001. She was a postdoctoral scholar at the Chemical Engineering Department of the University of California Santa Barbara (USA) from 2001-2003. She joined the University of Central Florida (USA) as an Assistant Professor of Physics in 2004, and became a full professor in 2012. From 2013-2017 she worked as Professor of Physics at the Ruhr University Bochum (Germany). She is the author of 200 peer-reviewed publications, 6 book chapters, and 6 patents. She serves in the editorial board of the Journal of Catalysis and the Chemical Reviews journal.
Beyond Static Models: Dynamics in Electrocatalysis
Climate change concerns have spurred a growing interest in developing environmentally friendly technologies for energy generation (i.e. green H2 from water splitting). Moreover, the electrochemical reduction of CO2 (CO2RR) into value-added chemicals offers an additional possibility to store renewable energy into chemical bonds. Thus, efficient, selective and durable catalysts must be developed. Nonetheless, in order to tailor the chemical reactivity of nanocatalysts, fundamental understanding of their structure and surface composition under reaction conditions must be obtained. It is by now well known that even morphologically and chemically well-defined pre-catalysts will be susceptible to drastic modifications during catalysis. Even more, such changes might be reversible when the applied external stimulus (e.g. electrical potential and/or chemical environment) is removed, resulting in misleading information on the nature of the active sites when only pre-natal and post-mortem characterization data are available.
This talk will provide examples of the operando evolution of catalysts employed CO2RR [1, 2] as well as the oxygen evolution reaction (OER) [3] using model pre-catalysts ranging from single atoms (Metal-N-C) to nanoparticles (Cu2O cubes, ZnO@Cu2O cubic NPs, CoOx NPs), to thin films (Co1+δFe2-δO4) and single crystals [Cu(100),(111),(310)]. Some of the aspects that will be discussed here include the understanding of the active state formation, and the correlation between the dynamically evolving structure and composition of the working catalysts and their catalytic performance. Our studies are expected to open up new routes for the reutilization of CO2 through its direct conversion into industrially valuable chemicals and fuels and a more efficient green hydrogen production.
Héctor D. Abruña
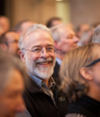
Dept. of Chemistry & Chemical Biology, Cornell University, Ithaca NY, USA
Professor Abruña, Émile M. Chamot Professor of Chemistry, is Director of the Center for Alkaline Based Energy Solutions (CABES), the Energy Materials Center at Cornell (emc2) and the Abruña Energy Initiative. He completed his graduate studies with Royce W. Murray and Thomas J. Meyer at the University of North Carolina at Chapel Hill in 1980 and was a postdoctoral research associate with Allen J. Bard at the University of Texas at Austin from 1980-81. After a brief stay at the University of Puerto Rico, he joined Cornell in 1983. He was Chair of the Department of Chemistry and Chemical Biology from 2004-2008. His research is focused on the development and operando characterization of energy materials for batteries, fuel cells and electrolyzers.
Prof. Abruña is a member of the National Academy of Sciences (2018), the American Academy of Arts and Sciences (2007) and Fellow of the American Association for the Advancement of Science (2007). He has been the recipient of numerous awards with the most recent ones including the A. J. Bard Award of the Electrochemical Society (2019) the Frumkin Medal of the International Society of Electrochemistry (2019) and the American Chemical Society Award in Analytical Chemistry (2021). Prof. Abruña is the co-author of over 570 publications (h-index = 105) and has given over 675 invited lectures world-wide. He considers his 65 Ph.D. students and 75 Post-Doctoral associates, to date, as his most important professional achievement.
Operando Methods for Mechanistic Studies of Energy Systems
This presentation will deal with the use of operando methods for mechanistic studies energy systems with emphasis on fuel cells and battery materials and technologies. The presentation will begin with a brief overview of the methods employed with emphasis on the use of X-ray based methods, transmission electron microscopy (TEM) under active potential control, confocal Raman spectroscopy and microscopy and DEMS (differential electrochemical mass spectrometry) coupled with SEIRAS (surface enhanced infra-red absorption spectroscopy). The utility of these methods will be illustrated by case studies focusing on non-precious metal electrocatalysts for the oxygen reduction reaction (ORR) in alkaline media, Li/S batteries the reduction of carbon dioxide and the oxidation of ethanol. The presentation will conclude with an assessment of future directions.
Heather J. Kulik
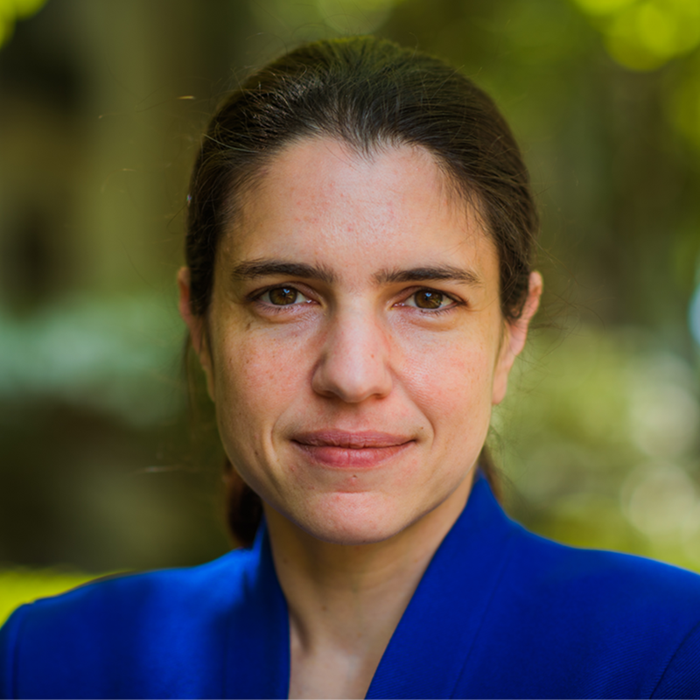
Massachusetts Institute of Technology, USA
Professor Heather J. Kulik is a tenured Associate Professor in the Departments of Chemical Engineering and Chemistry at MIT. She received her B.E. in Chemical Engineering from the Cooper Union in 2004 and her Ph.D. from the Department of Materials Science and Engineering at MIT in 2009. She completed postdoctoral training at Lawrence Livermore and Stanford, prior to joining MIT as a faculty member in November 2013. Her research has been recognized by an Office of Naval Research Young Investigator Award, DARPA Young Faculty Award and Director’s fellowship, NSF CAREER Award, a Sloan Fellowship in chemistry, a Distinguished Romberg Guest Professorship from the University of Heidelberg, and a Hans Fischer Senior Fellowship from the Technical University of Munich, among others.
Machine Learning for Discovery in Open Shell Transition Metal Catalysis
Overcoming the limits of approximate electronic structure models in machine learning accelerated materials discovery Machine learning (ML)-accelerated discovery of transition metal containing materials such as light-harvesting chromophores, phosphors, and other photoactive complexes holds great promise. Nevertheless, the open shell d electrons that impart many of the desirable properties to these systems also make them notoriously challenging to study with conventional electronic structure techniques such as density functional theory (DFT). Thus, when ML is used to accelerate computational screening, it often inherits the biases of the underlying method used to generate training data.
I will describe our recent efforts to overcome these limits through three complementary approaches. First, I will describe how we have developed machine learning models trained directly on experimental reports of iridium phosphors, leading to the development of ML models that can predict experimental emission energies and lifetimes with superior or equivalent performance to conventional methods such as time-dependent DFT but in a fraction of the computational time. Next, I will describe how we overcome limits of DFT uncertainty in screening for light harvesting chromophores with earth abundant 3d metals by incorporating method insensitivity into a multi-objective optimization workflow, requiring a consensus of functionals to agree on a property in order for a material to be selected as optimal. These workflows accelerate materials discovery by at least 1000-fold. Finally, I will describe our development of a density functional "recommender" that can identify which DFT functional is most predictive to obtain accurate vertical spin excitation energies in transition metal complexes.
Stefan Bernhard
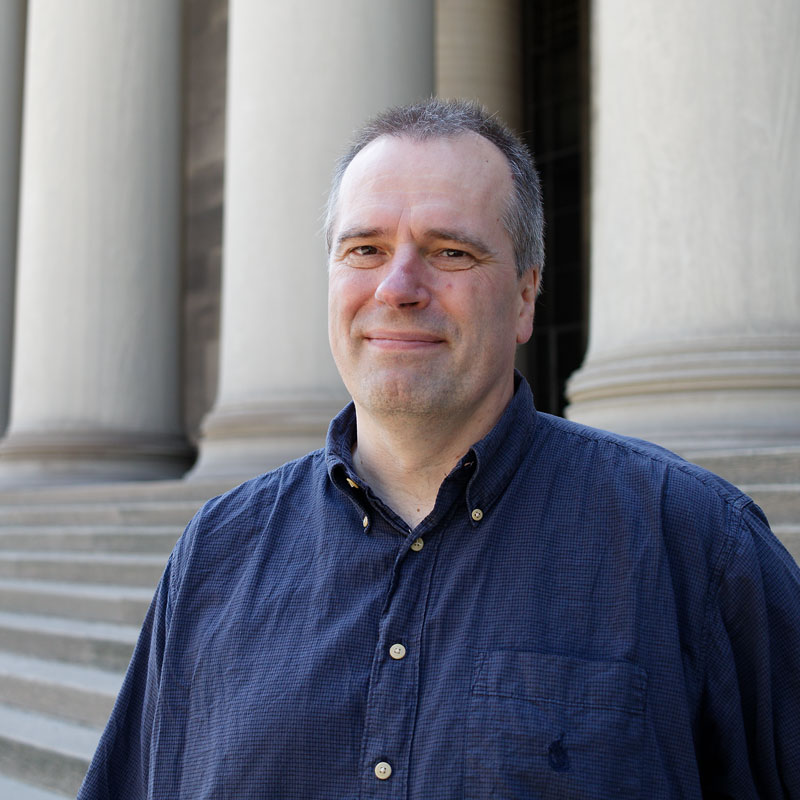
Department of Chemistry, Carnegie Mellon University, Pittsburgh PA, USA
Stefan Bernhard started his chemistry career as a laboratory technician with Chocolat Tobler, which was followed by a degree in chemical engineering from the Ingenieurschule Burgdorf. His undergraduate and graduate studies at the University of Fribourg were rewarded with a Ph.D. in 1996. These efforts were complemented by postdoctoral research in laser spectroscopy at Los Angeles National Laboratory and in the Abruña Group at Cornell University where work focused on electrochemical investigations. His first faculty appointment was at Princeton University where he started exploring luminescent metal complexes for optoelectronics and solar energy conversion applications. In 2009 he moved to Carnegie Mellon University where he now holds the rank of Professor of Chemistry.
Automated and Parallelized Discovery of Structure-Activity Relationships in Light-driven Chemical Reactions
This presentation features recently developed methods for investigating the complex interplay of reagents and catalysts in light-driven chemical processes relevant to solar fuel production. New 96-well photo-reactors were developed to massively parallelize and accelerate the quantification of photocatalytic rates and turnover numbers of photocatalytic alcohol reforming processes, photoredox transformations, and light-driven metal reductions. Reaction rates were measured using a camara-based detection system using indicator dyes and gas-sensitive films. Thousands of reaction conditions, catalysts, and chromophores were investigated with this new infrastructure built from single-board computers, 3-D printers, laser cutters and other techniques embraced by the Maker culture. Modern data science and machine learning techniques were used to pin-point trends in the structure-activity databases. A special focus was placed on the highly popular [Ir(C^N)2(N^N)]+ family of luminophores when libraries produced from cyclometalating C^N and 1,2-diimine N^N ligands created unprecedented numbers of structurally and electronically highly diverse photocatalyst structures [1]. Thousands of rates and turnover numbers for the photogeneration of hydrogen were measured using this library as documented in the figure below [2].
- Combinatorial syntheses allowed access to unprecedented structural diversity. Screening of these libraries of photosensitizers with highly diverse photo-physical properties offered unprecedented insights into the controlling structure/ activity relationships.
Sophia Haussener
Institute of Mechanical Engineering, EPFL, Lausanne, CH
Sophia Haussener is an Associate Professor heading the Laboratory of Renewable Energy Science and Engineering at EPFL. She received her PhD (2010) from ETH Zurich. Between 2011 and 2012, she was a postdoctoral researcher at the Joint Center of Artificial Photosynthesis (JCAP) and the Energy Environmental Technology Division of the Lawrence Berkeley National Laboratory (LBNL). She has published over 80 articles in peer-reviewed journals and conference proceedings, and 2 books. She has been awarded the ETH medal (2011), the Dimitris N. Chorafas Foundation award (2011), the ABB Forschungspreis (2012), the Prix Zonta (2015), the Global Change Award (2017), and the Raymond Viskanta Award on Radiative Transfer (2019), and is a recipient of the Starting Grant of the Swiss National Science Foundation (2014). She is a co-founder of the startup SoHHytec aiming at commercializing photoelectrochemical hydrogen production.
Photo-Electrochemical Fuel Generation: From Materials to Devices
The design and implementation of complete, operational photo-electrochemical devices requires a careful consideration of material requirements and their interplay in an integrated device. Multi-physical, multi-dimensional and multi-scale modeling tools are essential for the investigation of feasibility, optimization, and implementation of such devices. Modeling activities however always have to be fed and, ultimately, validated by experimental data of material and device characteristics. In this talk, I will discuss the development of predictive modeling tools for photo-electrochemical water and CO2 reduction. These models account for the atomistic-scale via density functional theory, molecular-scale by micro-kinetic and molecular dynamics models, nm-scale by double layer transport models, meso-scale by tomography-based direct numerical simulations, and device scale by volume-averaged device models. I will review each of these modeling steps and highlight the importance of interfacing them. I will then show what experimental data is needed to run these simulations and how these models can be validated. I will end with some device-level demonstrations of photo-electrochemical devices and highlight important differences when testing them in laboratory conditions and at on-sun conditions.